This article has been reviewed according to Science X's and . have highlighted the following attributes while ensuring the content's credibility:
fact-checked
peer-reviewed publication
trusted source
proofread
How the human–AI hybrid approach can lead to efficiency and effectiveness gains in marketing research
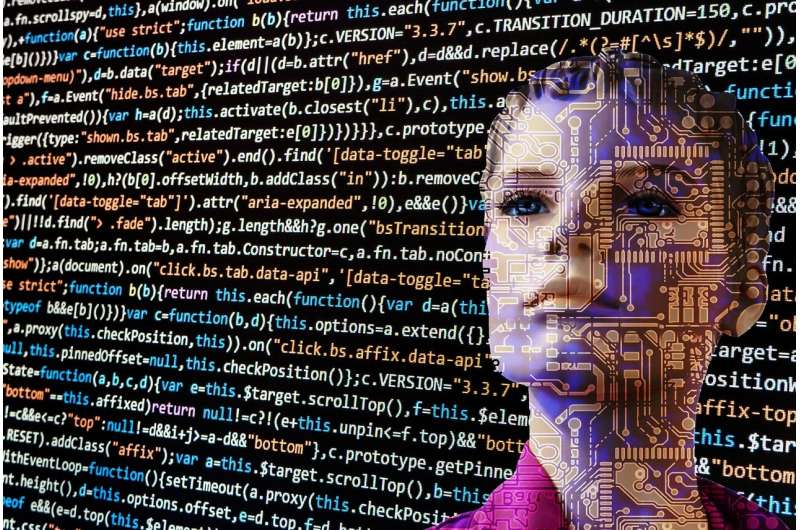
Researchers from University of Wisconsin-Madison have published a study that examines how a combination of Generative AI and human input leads to superior marketing research.
The study, in the Journal of Marketing, is titled "" and is authored by Neeraj Arora, Ishita Chakraborty, and Yohei Nishimura.
Generative AI (GenAI), and large language models (LLMs) in particular, are transforming marketing. According to a , over 70% of chief marketing officers have embraced this technology, and experts predict GenAI will revolutionize marketing research—a $84.3 billion dollar industry in 2023—by automating and enhancing data collection, analysis, and insights generation.
This new study finds that LLMs offer significant efficiency and effectiveness gains in the marketing research process for both qualitative and quantitative research. The researchers show that LLMs serve as excellent assistants for insights managers through different stages of the research process: study design, sample selection, data collection, and data analysis.
The AI–human hybrid approach
Consider a business context in which a brand manager collaborates with a consumer insights manager to formulate the problem the research is trying to address and come up with a set of research questions. The two may collaboratively agree on a research design that, for example, begins with exploratory research (e.g., in-depth interviews) followed by descriptive research (e.g., a survey).
These first two steps of the research process are largely led by humans. Although the brand and insight managers could consult an LLM to gather secondary research on the topic and explore use cases that could help inform the research questions or research design, they would still largely rely on their knowledge of the business context to formulate the research problem, questions, and design.
The central premise is that a human–LLM hybrid approach can lead to efficiency and effectiveness gains in the marketing research process. The researchers partnered with a Fortune 500 food company and replicated two studies the company had conducted using an LLM. The first study was qualitative and centered around business questions for the Friendsgiving celebration.
The second study focused on testing a new refrigerated dog food. Arora explains that "for each study we treated the original human studies as the 'ground truth' and benchmarked the LLM generated studies against them. This approach allowed us to objectively evaluate the quality of synthetic data and investigate the role LLMs could play in knowledge generation."
For qualitative research, the study indicates that LLMs are excellent assistants for data generation and analysis.
On the data generation front, LLMs effectively create desirable sample characteristics, generate synthetic respondents that match those characteristics, and conduct and moderate in-depth interviews. Results show that LLM-generated responses are superior in terms of depth and insightfulness.
On the analysis front, LLMs perform well, matching human experts in identifying key ideas, grouping them into themes, and summarizing information. Although LLMs missed some themes that humans detected, they generated some that humans did not. Expert judges find that human-LLM hybrids outperformed their human-only or LLM-only counterparts.
"The upshot is that LLMs and humans bring unique, complementary insights that managers should leverage," says Chakraborty.
A handy assistant
An LLM can be an excellent starting point for creating the first draft of a survey and can generate survey introductions, screener questions, and demographic questions with relative ease.
As Nishimura says, "the LLM can focus on the laborious, repetitive, and uninteresting tasks while the human expert can use this time savings to think more creatively about answers to the business questions and the quality of the insights."
A significant advantage of LLMs as an assistant is their low cost. This single factor will contribute toward rapid adoption of LLMs for insight generation. The gains here are likely to be higher for hard-to-reach respondents (e.g., doctors, senior managers) because synthetic respondents do not get tired and can provide lengthy answers to many questions.
In the B2B arena where the end users and buyers are not easy to reach, LLM could be quite helpful in supplementing the information gathered from human respondents. As an intelligent engine, an LLM could be a revolutionary generator of prior information for a wide variety of business questions at a low cost.
It is important to note that LLMs can be wrong, biased, or hallucinate when not trained on the relevant data. Therefore, a human supervisor is a necessary part of the marketing research knowledge production process. For example, a human can make decisions about when not to ask an LLM for help.
This could occur when the information sought is new not only to the company, but also to the world. Other examples include marketing research in cultural contexts to understand local customs and traditions, topics with ethical considerations such as targeting vulnerable populations, and obtaining insights from data containing personal information, where LLMs may lack the necessary safeguards for data security and privacy.
More information: Neeraj Arora et al, AI–Human Hybrids for Marketing Research: Leveraging Large Language Models (LLMs) as Collaborators, Journal of Marketing (2024).
Journal information: Journal of Marketing
Provided by American Marketing Association