This article has been reviewed according to Science X's and . have highlighted the following attributes while ensuring the content's credibility:
fact-checked
peer-reviewed publication
trusted source
proofread
A new tool for plant long non-coding RNA identification
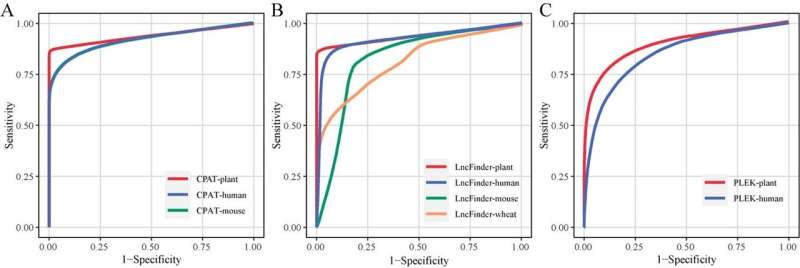
Long non-coding RNAs (lncRNAs) are ubiquitous transcripts with crucial regulatory roles in various biological processes, including chromatin remodeling, post-transcriptional regulation, and epigenetic modifications. While accumulating evidence elucidates mechanisms by which plant lncRNAs modulate growth, root development, and seed dormancy, their accurate identification remains challenging due to a lack of plant-specific methods.
Currently, the mainstream methods for plant lncRNA identification are largely developed based on human or animal datasets. Consequently, the accuracy and effectiveness of these methods in predicting plant lncRNAs has not been fully evaluated.
Recently, a research article titled "Plant-LncPipe: a computational pipeline providing significant improvement in plant lncRNA identification" by a group led by Jian-Feng Mao from Beijing Forestry University and Umeå University was in Horticulture Research.
This study extensively collected high-quality RNA-sequencing data from various plants and utilized these plant-specific data to retrain the models of three mainstream lncRNA prediction tools, namely CPAT, LncFinder, and PLEK. The performance of the retrained models was compared and evaluated against other popular lncRNA prediction tools, such as CPC2, CNCI, RNAplonc, and LncADeep.
The results demonstrated that the retrained models significantly improved the prediction performance for plant lncRNAs. Among them, two retrained models, LncFinder-plant and CPAT-plant, outperformed others on multiple evaluation metrics, rendering them the most suitable tools for plant lncRNA identification.
This research developed a computational pipeline named Plant-LncPipe for the identification and analysis of plant lncRNAs.
This pipeline integrates two top-performing identification models, CPAT-plant and LncFinder-plant, enabling a comprehensive computational process encompassing raw data preprocessing, transcript assembly, lncRNA identification, lncRNA classification, and lncRNA origins. This computational pipeline can be widely applied to various plant species. Plant-LncPipe is .
The study demonstrates that retraining lncRNA prediction models on high-quality plant transcriptomic data enabled more accurate capture of plant lncRNA features, significantly enhancing prediction precision and reliability. The study underscored the importance of species-specific retraining to improve model accuracy. Retraining existing mature models retained prior accumulated experience and methodologies while further boosting model applicability and accuracy.
More information: Xue-Chan Tian et al, Plant-LncPipe: a computational pipeline providing significant improvement in plant lncRNA identification, Horticulture Research (2024).
Journal information: Horticulture Research
Provided by Chinese Academy of Sciences