This article has been reviewed according to Science X's and . have highlighted the following attributes while ensuring the content's credibility:
fact-checked
trusted source
proofread
New study uses AI and machine learning to improve seasonal weather predictions
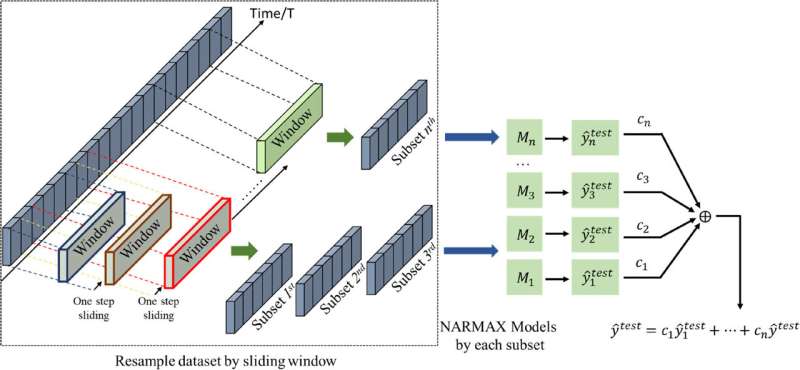
A team of researchers at the Universities of Lincoln, Sheffield, and Reading have developed a new method to improve the prediction of seasonal weather conditions in the U.K. and Northwest Europe.
The model offers a powerful tool in the quest to better understand changes in atmospheric circulation as well as make more accurate seasonal weather predictions. It could also benefit many sectors, including agri-food, energy, leisure, and tourism industries.
The study resulted in two published papers, one in and another in the .
To predict seasonal weather over Northwest Europe, major weather forecasting centers currently rely on expensive supercomputer models. To supplement these conventional methods, the group used an AI and machine learning method known as NARMAX (Nonlinear AutoRegressive Moving Average models with eXogenous inputs) to predict the state of the North Atlantic jet stream and atmospheric circulation, both of which are strongly linked to surface air temperature and precipitation anomalies.
NARMAX has been used successfully in many other fields of research, and in this case, early predictions were made for both summer and winter, for several different air circulation patterns that commonly affect the North Atlantic region and subsequent Northwest European seasonal weather.
The study results showed high accuracy for both seasons and all three circulation patterns examined. This is important because the conventional and more expensive supercomputer models struggle to accurately predict seasonal atmospheric conditions over this area in summer, tending to underestimate year-to-year variations for both seasons.
In addition, the NARMAX method has been used to analyze possible causes of atmospheric circulation changes. This information could be used for interpretation and to help improve the supercomputer model outputs.
This breakthrough could play a crucial role in improving seasonal forecasting, as well as informing the development of future weather forecasting models, particularly during the summer months.
Dr. Ian Simpson, Postdoctoral Research Associate at the University of Lincoln, commented, "We have demonstrated strong links between circulation and jet stream patterns and seasonal surface weather conditions in northwest Europe.
"Thus, having used NARMAX models to produce seasonal forecasts of circulation patterns, we can translate those into predictions of seasonal weather patterns, e.g., temperature and precipitation anomalies, in Northwest Europe that will be of interest to a diverse range of stakeholders.
"For example, providing more accurate seasonal forecasts will help the agri-food industry, helping to provide farmers with an idea of the likely yields for the season, and how best to optimize crop systems and plan for the harvest."
Edward Hanna, Professor of Climate Science and Meteorology at the University of Lincoln, added, "This is an exciting project that has brought together diverse disciplines and experts in meteorological science and machine learning with the aims of improving seasonal weather prediction and applying the results to end users.
"Our published papers demonstrate great potential for NARMAX modeling to play a significant role in helping to refine the next generation of supercomputer forecasting models, which have been historically computationally hungry, and in enhancing seasonal forecasts."
Dr. Yiming Sun, Research Associate at the University of Sheffield, said, "We have developed and applied a NARMAX machine learning method to predict the seasonal state of the North Atlantic atmospheric circulation and jet stream.
"The model has demonstrated a high degree of predictive accuracy compared to the dynamical models. Therefore, NARMAX can be used to help improve seasonal forecast skill and inform the development of dynamical supercomputer models."
More information: Yiming Sun et al, Probabilistic seasonal forecasts of North Atlantic atmospheric circulation using complex systems modelling and comparison with dynamical models, Meteorological Applications (2024).
Ian Simpson et al, North Atlantic atmospheric circulation indices: Links with summer and winter temperature and precipitation in north鈥恮est Europe, including persistence and variability, International Journal of Climatology (2024).
Provided by University of Lincoln